Data Quality Dashboard
Data quality refers to the overall utility, reliability, and accuracy of data for its intended use. High-quality data is essential for making informed decisions, improving business processes, and driving innovation. Poor data quality can lead to erroneous conclusions, missed opportunities, and operational inefficiencies.
How It Works
Data Collection
Data is collected from various sources, such as databases, spreadsheets, and external data providers. The quality of the source data significantly impacts the final data quality.
Data Profiling
The collected data is examined to understand its structure, content, and relationships. This includes identifying missing data, duplicates, and outliers.
Data Cleansing
Errors and inconsistencies in the data are corrected through processes such as filling missing values, removing duplicates, and standardizing formats.
Data Enrichment
External data is added to enhance the completeness and accuracy of existing data. For example, adding geolocation data to customer records.
Data Validation
The data is checked against predefined rules or business logic to ensure accuracy and consistency. This might include ensuring that certain fields are mandatory or that numerical values fall within an expected range.
Data Governance
Ongoing processes and policies ensure that data quality is maintained over time. This includes regular audits, data stewardship roles, and data management tools.
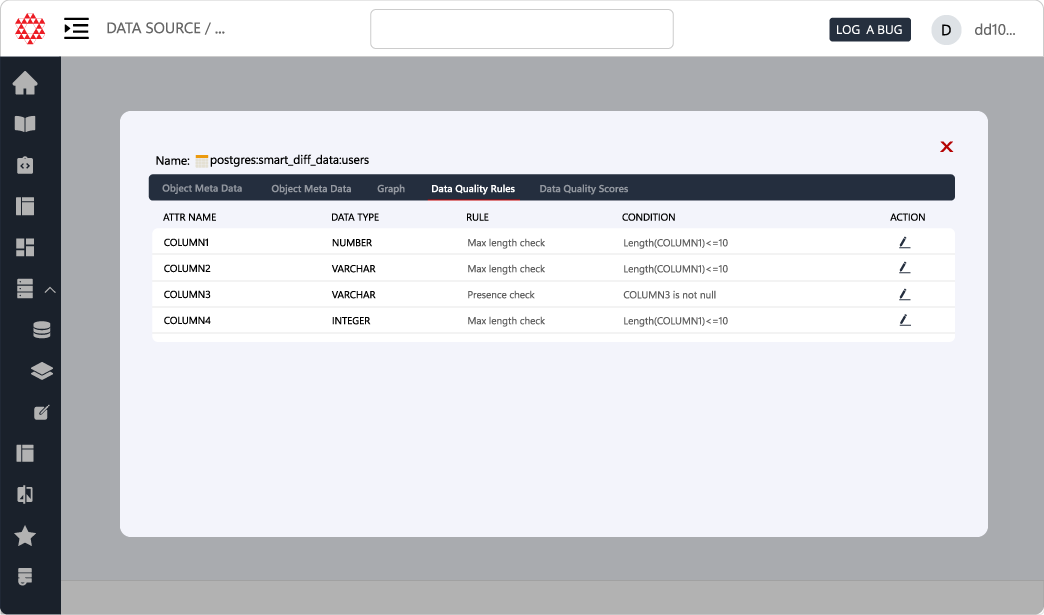
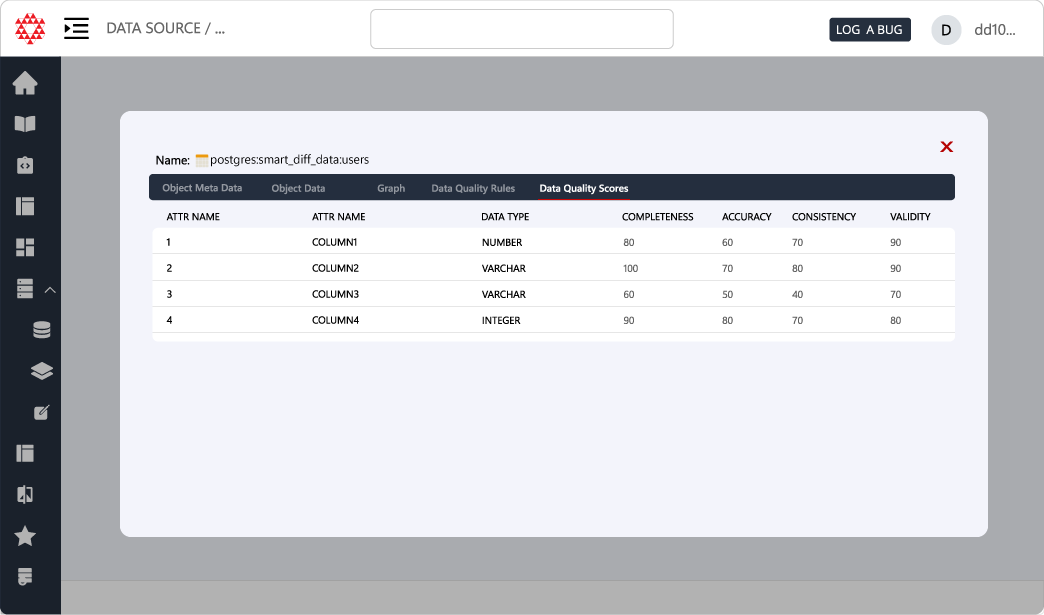
Benefits
Better Decision-Making
High-quality data allows organizations to make informed, data-driven decisions, reducing the risk of mistakes.
Operational Efficiency
Clean, well-organized data reduces inefficiencies and errors, improving productivity and resource allocation.
Increased Revenue
Accurate data can reveal opportunities for new revenue streams, improved customer satisfaction, and better-targeted marketing.
Regulatory Compliance
Many industries have strict regulations around data handling and reporting. High-quality data helps ensure compliance with these rules.
Enhanced Analytics
With reliable data, organizations can extract meaningful insights from analytics and machine learning models, leading to better innovation and competitive advantage.
Features
Accuracy
Data should reflect the real-world entities or events it describes. Any inaccuracies can lead to misinformed decisions.
Completeness
All required data should be present without gaps. Missing data can distort analysis and conclusions.
Consistency
Data should be consistent across different systems and datasets. For example, customer information should be identical in both the sales and support databases.
Timeliness
Data should be up-to-date to be relevant for decision-making. Outdated information can lead to incorrect conclusions.
Uniqueness
Data should not be duplicated unnecessarily. Duplicate records can cause confusion and errors.
Validity
Data should conform to defined formats and rules. For example, a date field should only contain valid dates, and an email field should only contain valid email addresses.
Accessibility
Data should be easily accessible to those who need it, while being protected from unauthorized access to ensure privacy and security.
By focusing on data quality, organizations can ensure that their data remains a valuable and trustworthy asset that drives success.